Attention
This website is best viewed in portrait mode.
Production optimization using digital twin - part 2
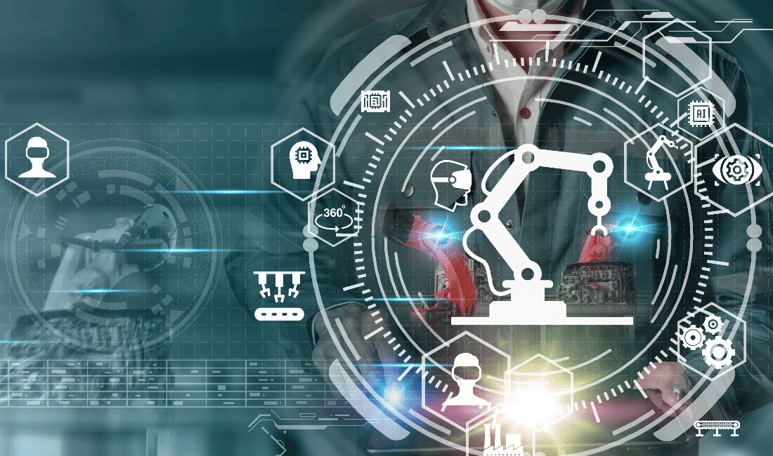
This is the second part of the blog on the impact of ESG criteria on the manufacturing sector. In the first blog, we saw how ESG criteria affect manufacturing and touched upon building an efficient plant is through virtual simulation. In this blog, we will see how to go about implementing virtual twins for production optimization along with a case study.
Author: Vishal Kamath, Senior Technical Manager, Advance Manufacturing Engineering
The current execution model
Let us look at how things are currently managed before we get into the specifics of digital twins and plant simulation. Many plants are designed using AutoCAD and Excel. This case will include senior resources who have previously built similar plants, as well as consultants such as system integrators, and there will be a lot of group discussion involving multi-disciplinary teams.
In these processes, many approximations are made, such as assuming the efficiency of a specific machine. Another disadvantage of this method is that the results are not visible until after the implementation and investments have been made. We need to have the plant built and ramp-up to complete production capacity to understand how the system performs.
This is where digital technologies like a simulation-based approach can help in the optimization of production systems. We can build a plant using a digital twin approach that allows us to run what-if scenarios well in advance, even before the first brick is laid.
GET IN TOUCH WITH US TO LEARN HOW SIMULATIONS CAN HELP YOU OPTIMIZE YOUR PROJECTS
A holistic approach to digital twin
For a comprehensive approach, we should consider three types of digital twins: product twins, production twins, and performance twins. So, what exactly is a product digital twin? A digital twin is a digital model that manages all of the geometry and product attributes. It is used in the creation and modification of a product. This is the most common type of digital twin that is used today and has been used for a long time in the form of CAD and CAE software such as CATIA, ANSYS, and others.
The other type is the production digital twin, which will be covered in greater detail in this blog. This is an established domain, and we've seen a wide range of simulation tools that assist manufacturers in planning, managing, and optimizing their operations in this space. And now, companies can capture actual production data and use it to drive and validate simulations, as well as continuously refine the production, thanks to IIoT and industry 4.0 practices.
Finally, there is the performance digital twin, which is now being used in very subtle ways because it is a logical extension to include product reliability data, quality data, and functional data as it is captured in services. The technology exists to monitor many products in service via Industrial Internet of Things (IIoT) strategies, but companies need to capture and then act upon this data.
Digital twin workflow
The process of creating the production digital twin can be classified as plant simulation or production simulation, and Let's take a holistic view of the simulation workflow. First, what are the inputs that go into creating a digital, digital twin, and what are the various types of scenarios that we can consider?
The inputs data is the same as what you need to build a plant which are preliminary layouts, process plans, standard operating procedures, preventive schedules, and setup time. Then, using data from the existing plant, we perform data verification.
CLICK HERE TO KNOW MORE ABOUT TATA ELXSI INDUSTRY 4.0 OFFERING
After completing the production model, we can run "what-if scenarios" to analyze the cycle time of a specific station. What if scenarios will investigate what happens when the cycle time of a specific station changes, as well as the effect of changing the buffer size. This throughput analysis is carried out for a set period, such as 15, 30, or 60 days, and identifies bottlenecks, where they will occur, and how to avoid them.
This will also help us determine the auxiliary system's requirements. What is the utilization of the plant or machine, what are the labour requirements, and how many operators with a specific skill set are needed? These are all obtained as outputs of the analysis.
Using digital twins, we can simulate the entire network, from material arrival from suppliers to how it is transported within the plant and to the stores, as well as the stores' production line and production line to final delivery. These simulations can be used for both greenfield and brownfield projects. This can be done during both the planning and execution phases. However, because there is no legacy data and a large number of system behaviours and identities must be evaluated during the planning phase, many iterations are required to optimize the solution.
GET IN TOUCH WITH US TO LEARN HOW SIMULATIONS CAN HELP YOU OPTIMIZE YOUR PROJECTS
Plant simulation for production optimization – example
The customer in question is a manufacturer in the consumer electronics industry. They were building a Greenfield project in India to produce wearables for the Indian and global markets. From the first stage to the final assembly, the manufacturing line had about 50 processes. We had to optimize the parameters using a baseline from another manufacturing plant that had been operational for a year.
Using Siemens Tecnomatix plant simulation software, we created models for each of the 50 processes and linked them according to the design process flow. The simulation model included parameters such as station availability, cycle time, setup time, technical availability (MTBF, MTTR) of stations, and material consumption data.
The simulation helped us determine the throughputs, bottleneck, lead-time, and efficiency and utilization of the machines and resources. Through the analysis and suggested optimizations, the customer was able to see, an 18% reduction in investments cost, 22% improvement in throughput and14% improvement in lead time.
CONTACT US TO FOR A FULL DETAILED CASE STUDY AND TO KNOW HOW WE CAN SUPPORT YOU