Attention
This website is best viewed in portrait mode.
Video Fingerprinting For Identification Of Counterfeit & Altered Media Content
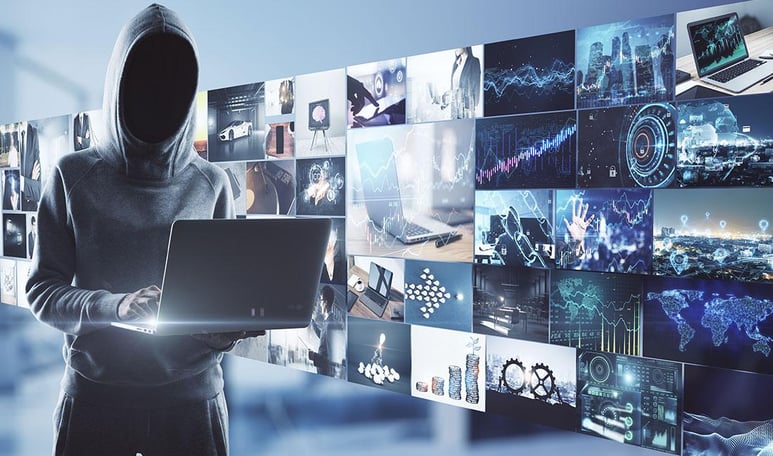
The evolution of media and broadcast technology has accelerated the production and distribution of multimedia content across various platforms. This has also catalyzed piracy efforts and created malicious, unwarranted content and its circulation over the internet. There is a surge of people surreptitiously recording movies, shows, sports, or other subscription content and using the internet to distribute it illegally.
Pirated video material gets over 230 billion views a year. Videos (films and TV shows) are the most pirated content on the internet, making up more than 66% of all pirated content. The movie industry alone is reported to incur a loss of around 40 billion dollars annually due to digital video piracy. Apart from economy and employment, video piracy also impacts licensing, which is a damaging issue as it stands as the backbone of the digital industry. There is an immediate need to curb such practices as it leads to the spread of misinformation.
Conventionally, watermarking techniques are used for copyright detection and management. Digital watermarking includes covertly embedding information related to the owner or distributor to help identify the source. But with sophistication in image processing methods, isolating watermarks and overwriting them with false information has become possible.
There are two major problems with the current methods. Firstly, watermarking aids in determining the original copyright owner of media, but it cannot determine the source of the altered content. Secondly, most pirated videos being circulated are recorded using cameras with low resolution and picture quality. These low-end cameras distort the watermarks and render them virtually useless.
Therefore, an efficient technique that isolates and decodes information from pirated content that reveals its source is required. This process should be applicable to all media content irrespective of the camera resolution of the device used to record it. It should be employable to any subscriber device, such as a set-top box, a smart television, a digital media player, a video game console, a mobile phone, a tablet, a vehicle infotainment system, or a laptop, without hampering user experience and video quality.
The key to identifying illegitimate copies of visual content being proliferated over social media or other streaming platforms is pointing out the origin of such content. While tracing the devices with access to specific content might be possible, it is nearly impossible to determine the source. To pinpoint the source, we need information linking content to users who access it. Such information is collected by the subscription systems, which have a list of users and the channels they subscribe to. Assuming that content a user is watching has been subscribed to by them, we can use subscriber-specific information such as the Media Access Control (MAC) address to create a link between media and the user.
We devised a system that would embed MAC addresses in images and videos as circular imprints corresponding to black-and-white binary images. The markers represent each value in a 12-bit MAC address in binary form.
The markers are designed to have low opacity and not interfere with the media's content. They are then superimposed over media content received from the broadcaster in a way that has minimal impact on its perception. Each marker is placed in a fixed location, depending on the media quality and dimensions.
To prevent the markers from being obfuscated by elements in the media or darker backgrounds in certain frames, embedding is done on most image frames. They are also indexed during generation to ensure retrieval and decoding even if the pirated video is captured at an angle or is rotated by 180 degrees.
Several permutations and combinations can be applied to the embedding process. Embedding asymmetrically would enable robust sequencing of a detected sub-code even when the unauthorized video is captured at different angles. We can vary the number of frames in which the markers are embedded or embed each marker to only a certain number of frames. We might also vary the marker shape altogether and include other colour patterns.
Embedding creates a link between the user and the media they access. On receiving an instance of suspected plagiarized media content, we first detect the presence of a marker using the Tag Detector. Neural Network architecture is used to locate markers present in images. The model was trained on images which varied in hue, brightness, quality, and overall content placement, which allowed it to be more versatile.
Once detected, the tags are further processed by the Tag Decoder. An object detection model is used to decode values from a tag. The model is trained to be robust to accurately decode each value regardless of resolution and content placement, which might impair most tags.
An alternative way to decode the model is using image processing techniques. Threshold values are calculated using pixel intensity which is further used to determine the value of each bit of the address.
The decoded values are averaged periodically to eliminate anomalous detections made due to diverging backgrounds of tags. Once all the frames are processed, the maximum of averaged values is chosen as the final decoded MAC address.
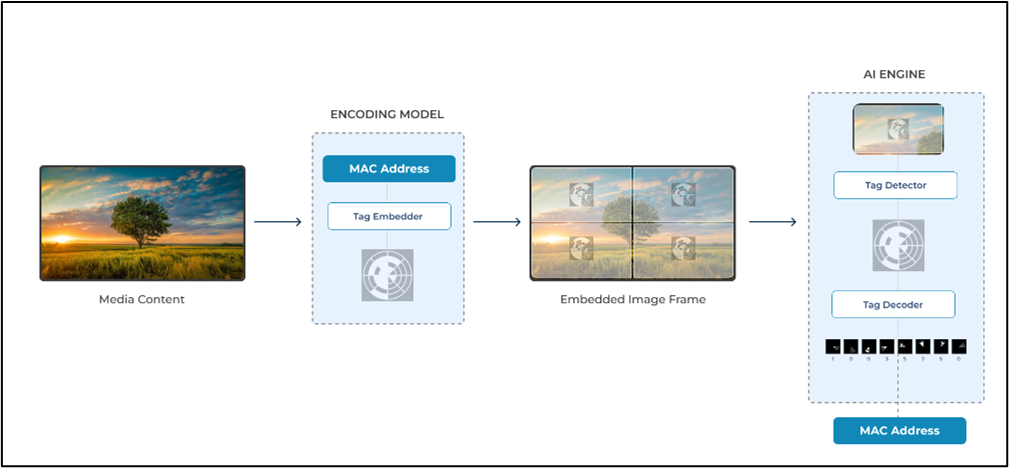
Schematic representation of the Encoding-Decoding process
Digital piracy adversely affects the media industry, including artists, creators, publishers, and distributors who spend a substantial amount of time, effort, and money to create content. The solution is a reliable method for precisely identifying the source of counterfeit media content. The system can correctly identify the source of the content with an accuracy of 90%. The embedding process has an almost negligible effect on the video quality and content. We ensure robust detection and well-founded results irrespective of differing backgrounds, brightness, and pixel intensity.
This method can easily be employed by set-top box satellite television companies, integrated with media players, portals of social media or at the back end of content hosting networks to analyze shared content and report authenticity to the broadcaster. It could have a large-scale impact on piracy.