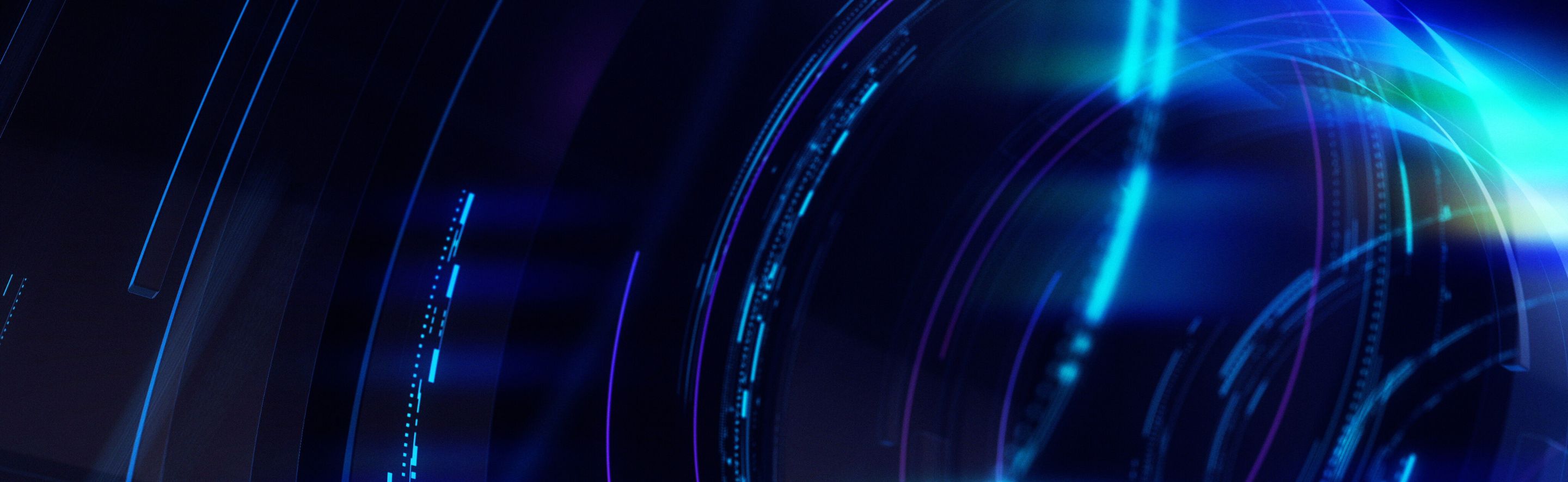
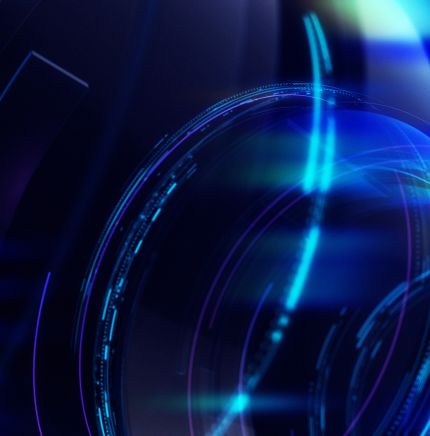
Blog
7 ways how AI is revolutionizing Medical Device Testing
Introduction
Artificial Intelligence and Machine Learning seem to be the new buzzwords of the 21st century. PwC, a professional services firm, predicts that AI will add $16 trillion to the global economy by 2030 while McKinsey puts the figure at $13 trillion. Sundar Pichai, CEO of Alphabet Inc and its subsidiary Google, has described developments in AI as “more profound than fire or electricity”. As Mr. Pichai’s comparison with electricity and fire suggests, AI and ML are general-purpose technologies capable of affecting entire economies. It excels in recognizing micro and macro patterns imperceptible to humans and can be very useful. Ever since the possibility of making machines learn by themselves came into existence, its applications have been used in almost every sector of the economy. The healthcare industry has been no exception.
AI's Expanding Role in Healthcare and Medical Device Testing
AI has been finding huge recognition in the field of medical diagnostics for the past few decades. However, one segment in the healthcare industry, that is relatively new to the use of AI is the verification and validation of medical devices. With the demands placed on testing and reliability towards delivery teams increasing exponentially over the years, it has become all the more important to take a step beyond just automation and start using AI and ML for medical device testing.
Verification and validation of medical devices is a long and non-trivial process that should be carried out simultaneously throughout the development process. Integrating AI and ML into this process can prove beneficial in many ways, and below are the seven such key areas that can get the most benefit out of it.
Key Benefits of Using AI in Medical Device Verification and Validation
1. Data-driven insights: As more and more data is being made available for mainstream processing and insight generation, decision science is now mostly driven by crafty usage of state-of-the-art AI and ML. Platforms and tools for medical device testing are becoming increasingly available to churn data in a short period and derive meaningful insights, making it available in near real-time. These AI tools can be used during product verification and validation to identify complex scenarios from the requirement traceability matrix.
2. Creating test cases: Test cases are usually designed by highly skilled test and automation engineers. This needs a combination of multi-disciplinary skills and collaborative effort across teams. By using AI tools, test cases can be generated automatically which takes multiple factors like functionality, scalability, coverage, loading into consideration. AI algorithm has the ability to look inside the code and call graphs to derive test cases that have a higher probability to unearth defect compared to the manual approach. The use of AI has led to a significant increase in the pace of test development. Intel’s homegrown AI technologies, CLIFF (Coverage LIFt Framework) and ITEM (Intelligent Test Execution Management) are a testament to how the use of AI can reduce the number of tests required in product validation by up to 70%.
3. Bringing intelligent automation to testing: Instead of running tests and fixing the bugs manually, AI-driven test controllers can be used to identify test case failures and run remediation steps (and also cover multiple regression cycles) in accordance with the type of fault detected. It helps to increase the automation coverage by approximately 30% when using AI.
4. Improving system agility: One of the primary reasons why automated tests fail is not for their lack of quality but the lack of their pace keeping with the changes that are taking place. AI-powered testing tools can be designed to learn from test data generated using the emerging MLOps process so that test automation systems can adapt quickly to system changes.
5. Self-healing capability: Testing is a continuous process in a medical device’s life cycle. Organizations spend around 15 to 25% of their time maintaining automated test cases. A self-heal capable system, driven by AI can be a great tool to reduce the burden on an ever-increasing testing budget as the system grows to be more and more complex. It is usually observed that about 60 to 70% of all defects reported can be addressed by employing AI-powered self-heal solution.
6. Minimizing manual labor: Manual testing of medical devices can be an arduous task as it involves several regulatory requirements. AI helps to reduce manual testing efforts at some steps by bringing cognitive functions using a combination of image and other sensors thereby improving the speed and accuracy of testing. It has been found that the use of AI in testing reduces maintenance costs by almost 40%.
7. Reducing bias: Quite often, automation engineers, comprising a small number of the development team, become a bottleneck since there is strong human bias involved as the same team is used for repeated tasks. The use of AI and ML effectively eliminates this bias for varying test cycles and products. The use of convolution-based deep neural networks for computer vision, transformer-based networks for natural language processing, and other customized versions of perceptrons ensembled in a layered stack have the potential to mimic a complex cognitive process required for the task. This not only helps to bring raw machine power to solve problems once thought to be in the realm of human dexterity but also brings a new diversity which reduces the bias involving humans.
Challenges in Applying AI to Medical Device Testing
Although AI provides numerous benefits for medical device testing, it should be noted that the use of AI and ML also comes with some unique challenges. The first one is a practical challenge about the use of the right dataset. The machine-learning revolution has been built on improved algorithms, powerful computers to run these algorithms, and data from which they can learn. Yet data is not always readily available. Even when data exist, they can contain hidden assumptions that can be confusing for a machine. Moreover, the newest AI systems' demand for computing power can be expensive.
The second challenge is that AI and ML are powerful pattern-recognition tools, but lack many finer cognitive abilities that we humans take for granted. It generalizes from the rules it discovers, sometimes excelling at well-bounded tasks, but can get things wrong if confronted with unexpected input.
The use of AI and ML in medical device testing has its share of pros and cons, however, its benefits far outweigh some of the challenges associated with it.